Pathways to identify and reduce uncertainties in agricultural climate impact assessments
Both climate and impact models are essential for understanding and quantifying the impact of climate change on agricultural productivity. Multi-model ensembles have highlighted considerable uncertainties in these assessments, yet a systematic approach to quantify these uncertainties is lacking. We propose a standardized approach to attribute uncertainties in multi-model ensemble studies, based on insights from the Agricultural Model Intercomparison and Improvement Project. We find that crop model processes are the primary source of uncertainty in agricultural projections (over 50%), excluding unquantified hidden uncertainty that is not explicitly measured within the analyses. We propose multidimensional pathways to reduce uncertainty in climate change impact assessments.
This is a preview of subscription content, access via your institution
Access options
Access Nature and 54 other Nature Portfolio journals
Get Nature+, our best-value online-access subscription
cancel any time
Subscribe to this journal
Receive 12 digital issues and online access to articles
133,45 € per year
only 11,12 € per issue
Buy this article
- Purchase on SpringerLink
- Instant access to full article PDF
Prices may be subject to local taxes which are calculated during checkout
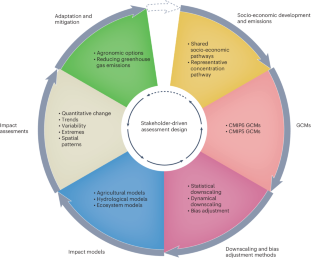
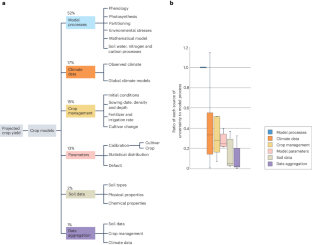
Similar content being viewed by others
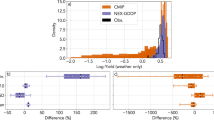
Statistically bias-corrected and downscaled climate models underestimate the adverse effects of extreme heat on U.S. maize yields
Article Open access 20 September 2021
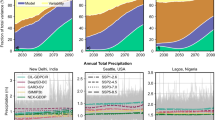
Downscaling and bias-correction contribute considerable uncertainty to local climate projections in CMIP6
Article Open access 30 September 2023
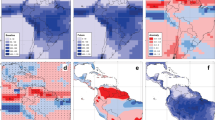
High-resolution and bias-corrected CMIP5 projections for climate change impact assessments
Article Open access 20 January 2020
References
- Bezner Kerr, R. et al. in Climate Change 2022: Impacts, Adaptation and Vulnerability(eds Pörtner, H.-O. et al.) 713–906 (Cambridge Univ. Press, 2022).
- IPCC. Climate Change 2023: Synthesis Report (eds Lee, H. et al.) 35–115 (IPCC, 2023).
- Wang, B. et al. Sources of uncertainty for wheat yield projections under future climate are site-specific. Nat. Food1, 720–728 (2020). ArticleCASPubMedGoogle Scholar
- Jägermeyr, J. et al. Climate impacts on global agriculture emerge earlier in new generation of climate and crop models. Nat. Food2, 873–885 (2021). ArticlePubMedGoogle Scholar
- Xiong, W. et al. Different uncertainty distribution between high and low latitudes in modelling warming impacts on wheat. Nat. Food1, 63–69 (2020). ArticleGoogle Scholar
- Rosenzweig, C. et al. Assessing agricultural risks of climate change in the 21st century in a global gridded crop model intercomparison. Proc. Natl Acad. Sci. USA111, 3268 (2014). ArticleADSCASPubMedGoogle Scholar
- Asseng, S. et al. Uncertainty in simulating wheat yields under climate change. Nat. Clim. Change3, 827–832 (2013). ArticleADSCASGoogle Scholar
- Knutti, R., Furrer, R., Tebaldi, C., Cermak, J. & Meehl, G. A. Challenges in combining projections from multiple climate models. J. Clim.23, 2739–2758 (2010). ArticleADSGoogle Scholar
- Rosenzweig, C. et al. The Agricultural Model Intercomparison and Improvement Project (AgMIP): protocols and pilot studies. Agric. For. Meteorol.170, 166–182 (2013). ArticleADSGoogle Scholar
- Wallach, D., Mearns, L. O., Ruane, A. C., Rötter, R. P. & Asseng, S. Lessons from climate modeling on the design and use of ensembles for crop modeling. Clim. Change139, 551–564 (2016). ArticleADSPubMedPubMed CentralGoogle Scholar
- Wallach, D. et al. Multimodel ensembles improve predictions of crop–environment–management interactions. Glob. Change Biol.24, 5072–5083 (2018). ArticleADSGoogle Scholar
- Martre, P. et al. Multimodel ensembles of wheat growth: many models are better than one. Glob. Change Biol.21, 911–925 (2015). ArticleADSGoogle Scholar
- Falloon, P. et al. Ensembles and uncertainty in climate change impacts. Front. Environ. Sci.2, 33 (2014).
- Ruane, A. C. et al. Biophysical and economic implications for agriculture of +1.5° and +2.0 °C global warming using AgMIP Coordinated Global and Regional Assessments. Clim. Res.76, 17–39 (2018). ArticleADSPubMedPubMed CentralGoogle Scholar
- Cammarano, D. et al. Uncertainty of wheat water use: simulated patterns and sensitivity to temperature and CO2. Field Crops Res.198, 80–92 (2016). ArticleGoogle Scholar
- Toreti, A. et al. Narrowing uncertainties in the effects of elevated CO2 on crops. Nat. Food1, 775–782 (2020). ArticleCASPubMedGoogle Scholar
- O’Leary, G. J. et al. Response of wheat growth, grain yield and water use to elevated CO2 under a Free-Air CO2 Enrichment (FACE) experiment and modelling in a semi-arid environment. Glob. Change Biol.21, 2670–2686 (2015). ArticleADSGoogle Scholar
- Gao, Y. et al. Comparison of three calibration methods for modeling rice phenology. Agric. For. Meteorol.280, 107785 (2020). ArticleGoogle Scholar
- Warszawski, L. et al. The Inter-Sectoral Impact Model Intercomparison Project (ISI–MIP): project framework. Proc. Natl Acad. Sci. USA111, 3228–3232 (2014). ArticleADSCASPubMedGoogle Scholar
- Asseng, S. et al. Climate Change impact and adaptation for wheat protein. Glob. Change Biol.25, 155–173 (2019). ArticleADSGoogle Scholar
- Ruane, A. C. et al. Climate change impact uncertainties for maize in Panama: farm information, climate projections, and yield sensitivities. Agric. For. Meteorol.170, 132–145 (2013). ArticleADSGoogle Scholar
- Ruane, A. C. et al. Strong regional influence of climatic forcing datasets on global crop model ensembles. Agric. For. Meteorol.300, 108313 (2021). ArticleGoogle Scholar
- Maiorano, A. et al. Crop model improvement reduces the uncertainty of the response to temperature of multi-model ensembles. Field Crops Res.202, 5–20 (2017). ArticleGoogle Scholar
- Sun, T. et al. Current rice models underestimate yield losses from short-term heat stresses. Glob. Change Biol.27, 402–416 (2021). ArticleADSCASGoogle Scholar
- Wallach, D. et al. Accounting for both parameter and model structure uncertainty in crop model predictions of phenology: a case study on rice. Eur. J. Agron.88, 53–62 (2017). ArticleGoogle Scholar
- Ruane, A. C. et al. The Climatic Impact-Driver Framework for assessment of risk-relevant climate information. Earths Future10, e2022EF002803 (2022). ArticleADSPubMedPubMed CentralGoogle Scholar
- Clark, M. P. et al. Characterizing uncertainty of the hydrologic impacts of climate change. Curr. Clim. Change Rep.2, 55–64 (2016). ArticleGoogle Scholar
- Bosshard, T. et al. Quantifying uncertainty sources in an ensemble of hydrological climate-impact projections. Water Resour. Res.49, 1523–1536 (2013). ArticleADSGoogle Scholar
- Thuiller, W., Guéguen, M., Renaud, J., Karger, D. N. & Zimmermann, N. E. Uncertainty in ensembles of global biodiversity scenarios. Nat. Commun.10, 1446 (2019). ArticleADSPubMedPubMed CentralGoogle Scholar
- Aryal, A., Shrestha, S. & Babel, M. S. Quantifying the sources of uncertainty in an ensemble of hydrological climate-impact projections. Theor. Appl. Climatol.135, 193–209 (2019). ArticleADSGoogle Scholar
- Nishina, K. et al. Decomposing uncertainties in the future terrestrial carbon budget associated with emission scenarios, climate projections, and ecosystem simulations using the ISI-MIP results. Earth Syst. Dynam.6, 435–445 (2015). ArticleADSGoogle Scholar
- Brodie, S. et al. Recommendations for quantifying and reducing uncertainty in climate projections of species distributions. Glob. Change Biol.28, 6586–6601 (2022). ArticleCASGoogle Scholar
- Franke, J. A. et al. The GGCMI Phase 2 experiment: global gridded crop model simulations under uniform changes in CO2, temperature, water, and nitrogen levels (protocol version 1.0). Geosci. Model Dev.13, 2315–2336 (2020). ArticleADSGoogle Scholar
- Liu, K. et al. Silver lining to a climate crisis in multiple prospects for alleviating crop waterlogging under future climates. Nat. Commun.14, 765 (2023). ArticleADSCASPubMedPubMed CentralGoogle Scholar
- Xiong, W. et al. Calibration-induced uncertainty of the EPIC model to estimate climate change impact on global maize yield. J. Adv. Model. Earth Syst.8, 1358–1375 (2016). ArticleADSGoogle Scholar
- Amroun, H., Hafid, F. & Mehdi, A. How statistical modeling and machine learning could help in the calibration of numerical simulation and fluid mechanics models? Application to the calibration of models reproducing the vibratory behavior of an overhead line conductor. Array15, 100187 (2022). ArticleGoogle Scholar
- Valdivia, R. O. et al. in Handbook of Climate Change and Agroecosystems: The Agricultural Model Intercomparison and Improvement Project (AgMIP) Integrated Crop and Economic Assessments, Part 1 (eds Rosenzweig, C. & Hillel, D.) 101–156 (Imperial College Press, 2015).
- Zhang, S., Tao, F. & Zhang, Z. Uncertainty from model structure is larger than that from model parameters in simulating rice phenology in China. Eur. J. Agron.87, 30–39 (2017). ArticleGoogle Scholar
- Hasegawa, T. et al. Causes of variation among rice models in yield response to CO2 examined with free-air CO2 enrichment and growth chamber experiments. Sci. Rep.7, 14858 (2017). ArticleADSPubMedPubMed CentralGoogle Scholar
- Bassu, S. et al. How do various maize crop models vary in their responses to climate change factors? Glob. Change Biol.20, 2301–2320 (2014). ArticleADSGoogle Scholar
- Sándor, R. et al. Ensemble modelling of carbon fluxes in grasslands and croplands. Field Crops Res.252, 107791 (2020). ArticleGoogle Scholar
- Webber, H. et al. Diverging importance of drought stress for maize and winter wheat in Europe. Nat. Commun.9, 4249 (2018). ArticleADSPubMedPubMed CentralGoogle Scholar
- Wallach, D. et al. Proposal and extensive test of a calibration protocol for crop phenology models. Agron. Sustainable Dev.43, 46 (2023). ArticleGoogle Scholar
- Gao, Y. et al. Evaluation of crop model prediction and uncertainty using Bayesian parameter estimation and Bayesian model averaging. Agric. For. Meteorol.311, 108686 (2021). ArticleGoogle Scholar
- Folberth, C. et al. Uncertainty in soil data can outweigh climate impact signals in global crop yield simulations. Nat. Commun.7, 11872 (2016). ArticleADSCASPubMedPubMed CentralGoogle Scholar
- Wang, B., Liu, D. L., Waters, C. & Yu, Q. Quantifying sources of uncertainty in projected wheat yield changes under climate change in eastern Australia. Clim. Change151, 259–273 (2018).
- Huang, M. et al. Dominant sources of uncertainty in simulating maize adaptation under future climate scenarios in China. Agric. Syst.199, 103411 (2022). ArticleGoogle Scholar
- Dueri, S. et al. Simulation of winter wheat response to variable sowing dates and densities in a high-yielding environment. J. Exp. Bot.73, 5715–5729 (2022). ArticlePubMedPubMed CentralGoogle Scholar
- Kamali, B. et al. Uncertainty in climate change impact studies for irrigated maize cropping systems in southern Spain. Sci. Rep.12, 4049 (2022). ArticleADSCASPubMedPubMed CentralGoogle Scholar
- Constantin, J. et al. Management and spatial resolution effects on yield and water balance at regional scale in crop models. Agric. For. Meteorol.275, 184–195 (2019). ArticleADSGoogle Scholar
- Tao, F. et al. Contribution of crop model structure, parameters and climate projections to uncertainty in climate change impact assessments. Glob. Change Biol.24, 1291–1307 (2018). ArticleADSGoogle Scholar
- Jiang, T. et al. Identifying sources of uncertainty in wheat production projections with consideration of crop climatic suitability under future climate. Agric. For. Meteorol.319, 108933 (2022). ArticleGoogle Scholar
- Hoffmann, H. et al. Impact of spatial soil and climate input data aggregation on regional yield simulations. PLoS ONE11, e0151782 (2016). ArticlePubMedPubMed CentralGoogle Scholar
- Zhao, G. et al. Effect of weather data aggregation on regional crop simulation for different crops, production conditions, and response variables. Clim. Res.65, 141–157 (2015). ArticleGoogle Scholar
Acknowledgements
This work supported G.J.O’L. in the AGFACE project that was funded by the Australian Grains Research and Development Corporation and the Australian Government Department of Agriculture in a collaboration between Agriculture Victoria and the University of Melbourne. The contributions of A.C.R. were made possible by support from the NASA Earth Science Division to the GISS Climate Impacts Group.
Author information
Authors and Affiliations
- New South Wales Department of Primary Industries, Wagga Wagga Agricultural Institute, Wagga Wagga, New South Wales, Australia Bin Wang, Linchao Li & De Li Liu
- Gulbali Institute for Agriculture, Water and Environment, Charles Sturt University, Wagga Wagga, New South Wales, Australia Bin Wang & De Li Liu
- NASA Goddard Institute for Space Studies, New York, NY, USA Jonas Jägermeyr, Alex C. Ruane & Cynthia Rosenzweig
- Columbia University, Climate School, New York, NY, USA Jonas Jägermeyr
- Potsdam Institute for Climate Impacts Research, Member of the Leibniz Association, Potsdam, Germany Jonas Jägermeyr
- Agriculture Victoria, Department of Energy, Environment and Climate Action, Horsham, Victoria, Australia Garry J. O’Leary
- Faculty of Science, The University of Melbourne, Parkville, Victoria, Australia Garry J. O’Leary
- Institute of Crop Science and Resource Conservation, University of Bonn, Bonn, Germany Daniel Wallach
- College of Land Science and Technology, China Agricultural University, Beijing, China Puyu Feng
- State Key Laboratory of Soil Erosion and Dryland Farming on the Loess Plateau, Institute of Soil and Water Conservation, Northwest A&F University, Yangling, China Linchao Li & Qiang Yu
- Climate Change Research Centre, University of New South Wales, Sydney, New South Wales, Australia De Li Liu
- GreenCollar, The Rocks, Sydney, New South Wales, Australia Cathy Waters
- Technical University of Munich, School of Life Sciences, Digital Agriculture, HEF World Agricultural Systems Center, Freising, Germany Senthold Asseng
- Bin Wang